What is Qumulo?
Qumulo’s Scale Anywhere™ platform is a 100% software solution for hybrid enterprises to efficiently store and manage file & object data at the edge, in the core, and in the cloud.
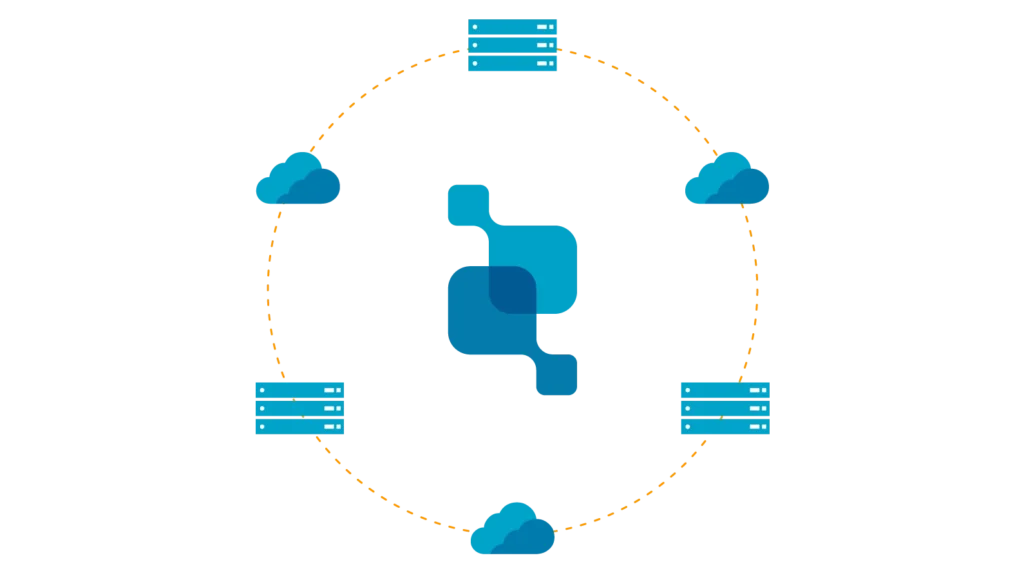
Why Scale Anywhere™?
Your business is creating and consuming more data, in more places, with workflows and workforces more geographically dispersed than ever before. As your data grows and spreads across locations, data silos create:
Spiraling costs
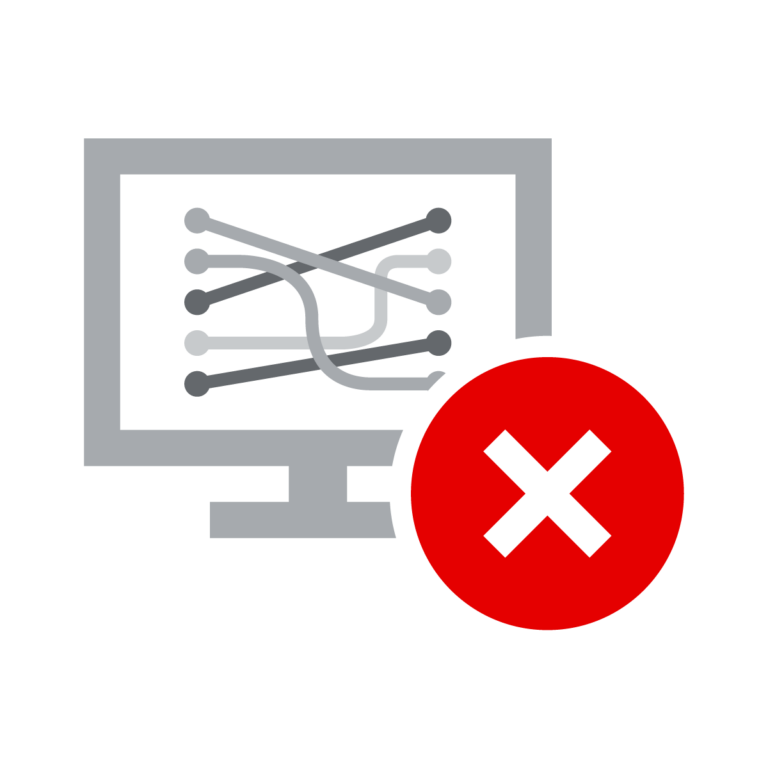
Management complexity
Weakened security
Reduced business agility
Your legacy storage platforms are not enough anymore. You need a unified un structured data platform that can run and scale everywhere data is created, stored, and accessed.
One platform, all unstructured data, everywhere
Qumulo eliminates the chaos of managing different platforms for your on-prem, cloud, high-performance, and archive workflows. The world’s first Scale Anywhere platform delivers a single architecture that can power all your exabyte-scale, geographically-distributed, multi-site, and multi-cloud data storage needs.
Boost Agility
Unify data access on a common platform to simplify collaboration between globally distributed teams.
Accelerate innovation
Leverage the speed and simplicity of the cloud to innovate more and manage less.
Reduce costs
Minimize operational overhead with predictable OpEx for all deployments, whether on-prem or in the cloud.
File in the cloud with no compromise
Azure Native Qumulo is the world’s first exabyte-scale, enterprise-grade, fully-managed file service in the cloud that enables truly elastic performance and capacity on demand – at a price point that can match or even beat your on-premises NAS. No re-platforming. No feature compromise. Predictable consumption-based pricing and available in minutes.
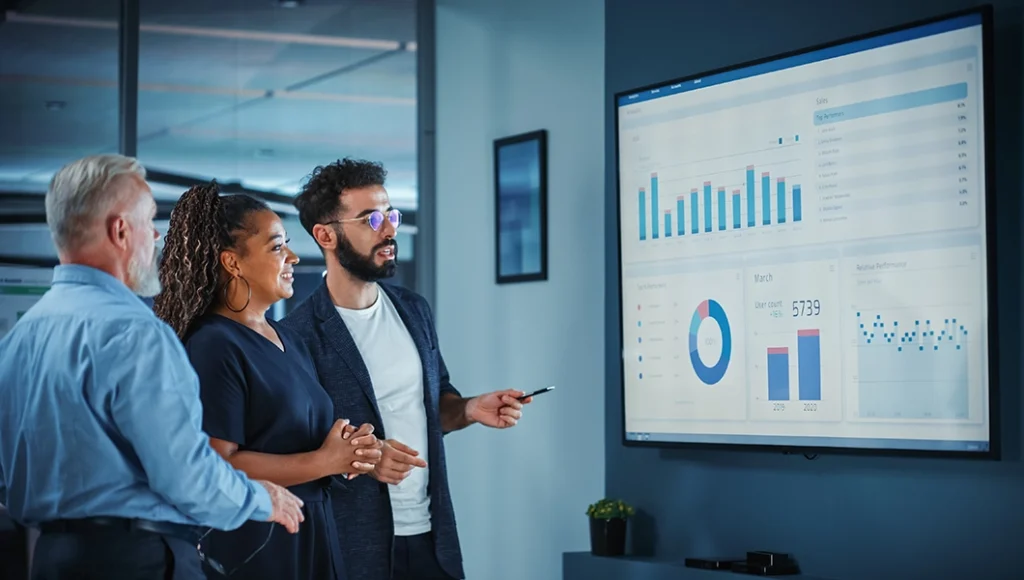
Unbeatable economics
- Almost 80% less expensive than the nearest alternative
- Comparable TCO to that of on-premises NAS
- Consumption-based pricing, metered to that minute
- Compare Costs
Elastic Performance
- 1GBps of sustained throughput included
- Pay only for additional throughput used, only while you use it
- Scale performance and capacity independently
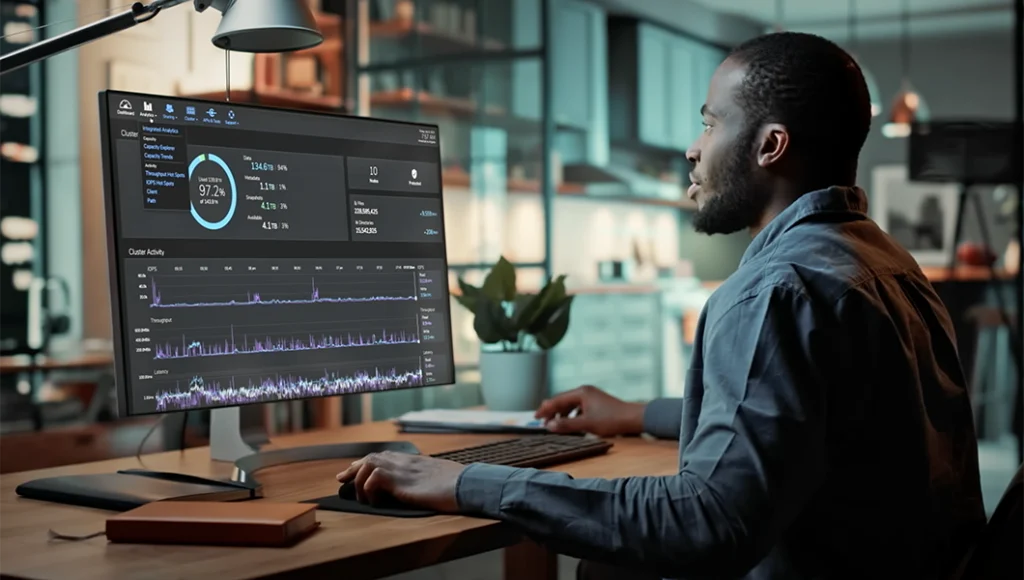
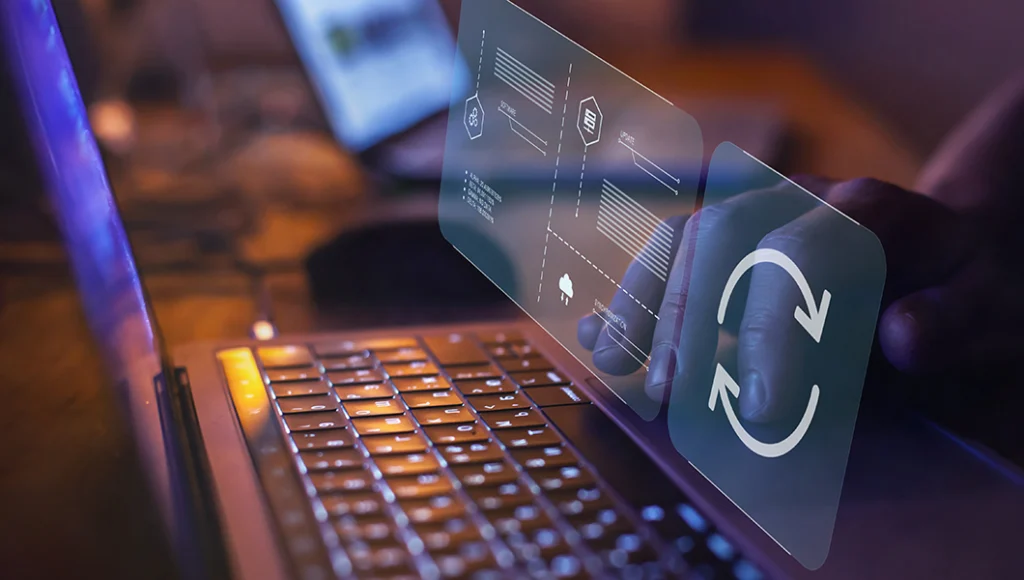
Unparalleled Data Durability
- All data is inherently protected
- 12 nines of data durability
- Based on Zone Redundant Storage (Azure Blob Block)
- Eliminates the need for on-prem redundancy
Cloud Speed and Simplicity
- Provision in minutes, not weeks or months
- Scale up and down on the fly as capacity and throughput demands change
- No need for expensive, risky re-platforming
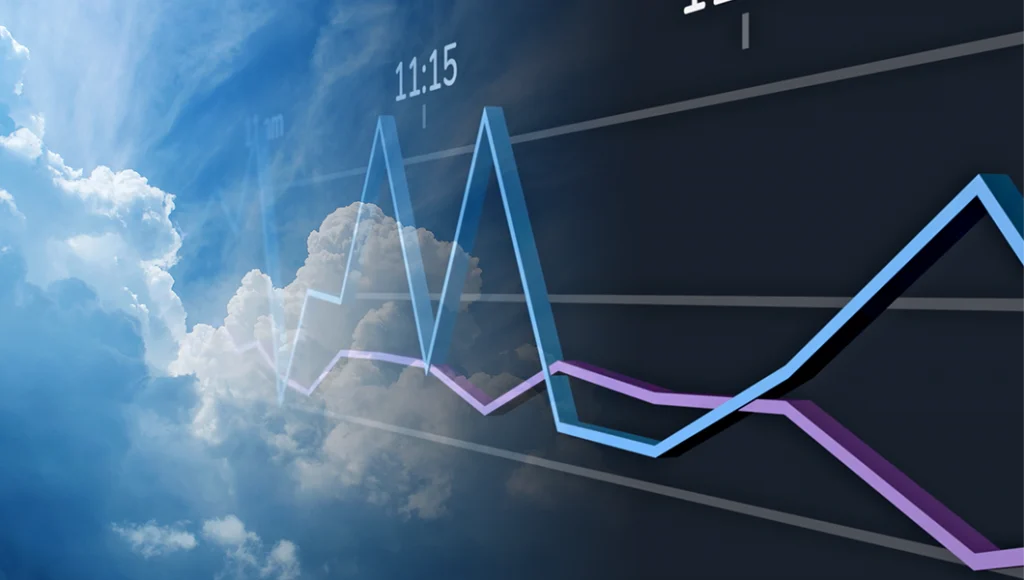
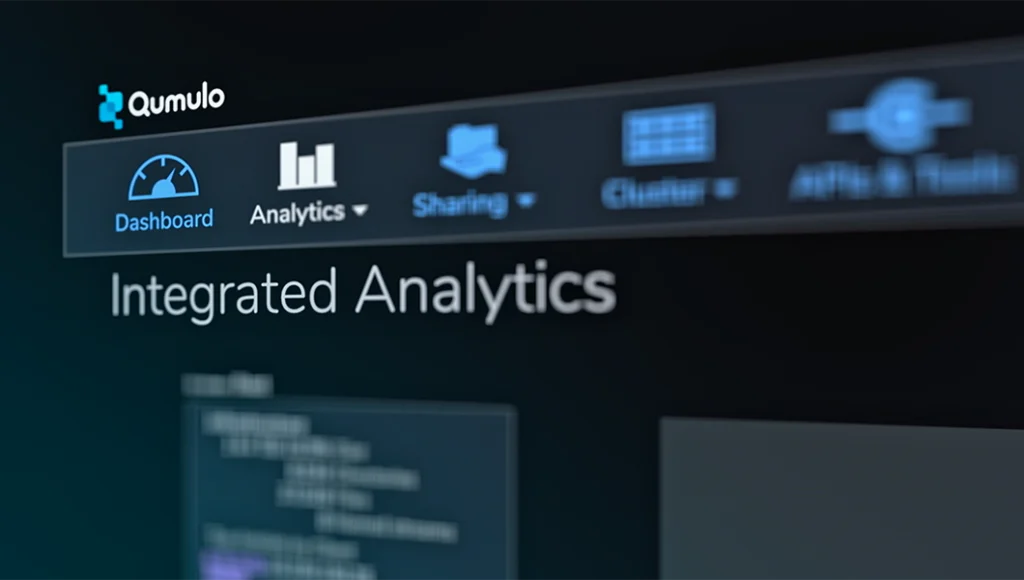
Rich Enterprise Data Services
- Full multiprotocol (NFS, SMB, S3, and more)
- Quotas, snapshots, continuous replication
- Real-time data visibility and analytics
- Rich API stack to automate operations
The power of One: Data simplified at scale
You have more data in more locations, with more people needing access across increasingly hybridized workflows. Here’s why Qumulo is the only platform fit for the modern enterprise.
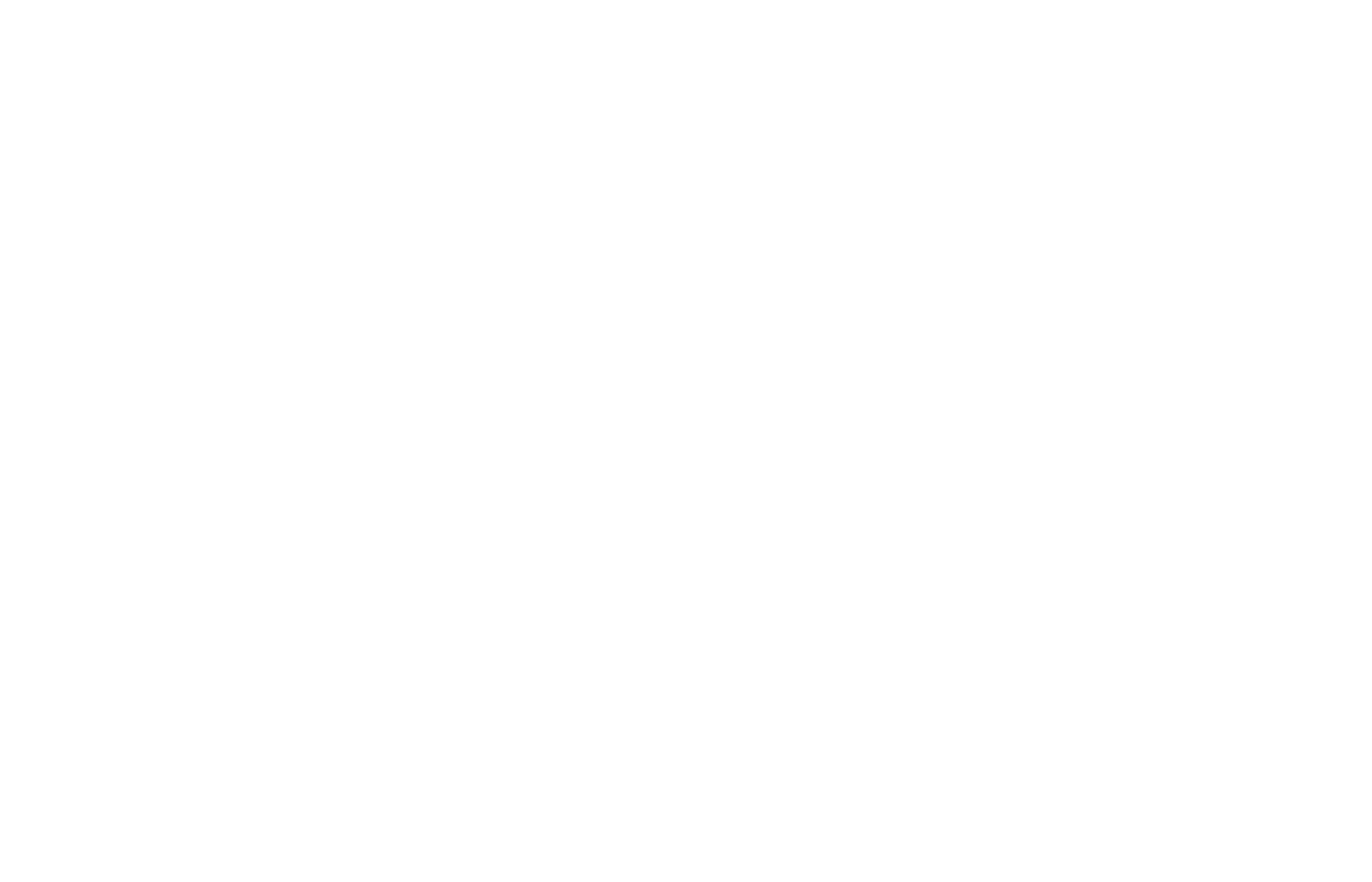
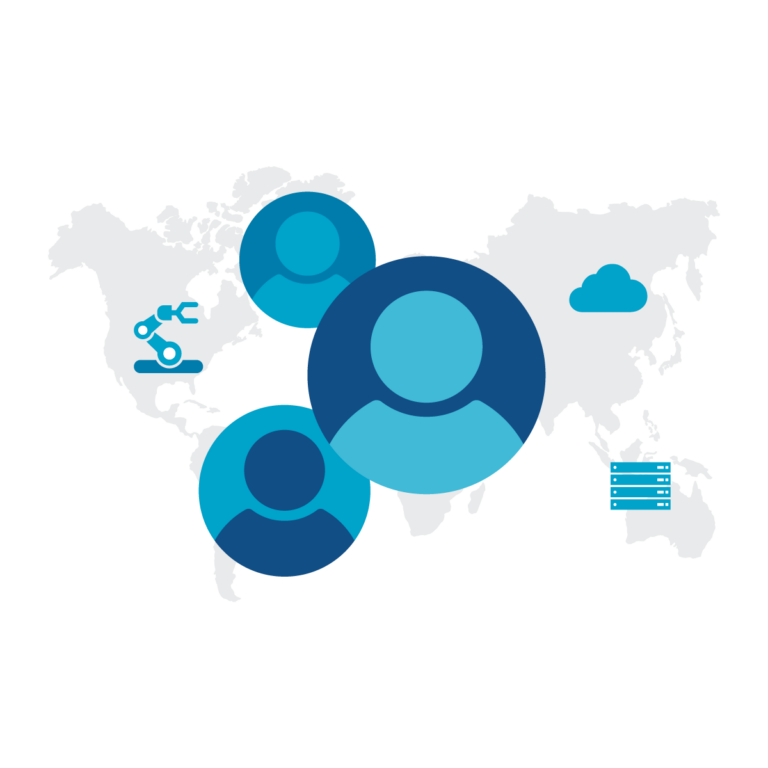
One Global Namespace
Qumulo-Global Namespace (Q-GNS) enables enterprises to access remote data as if it were local for all their workflows, from the most performance-sensitive apps to long-term archives.
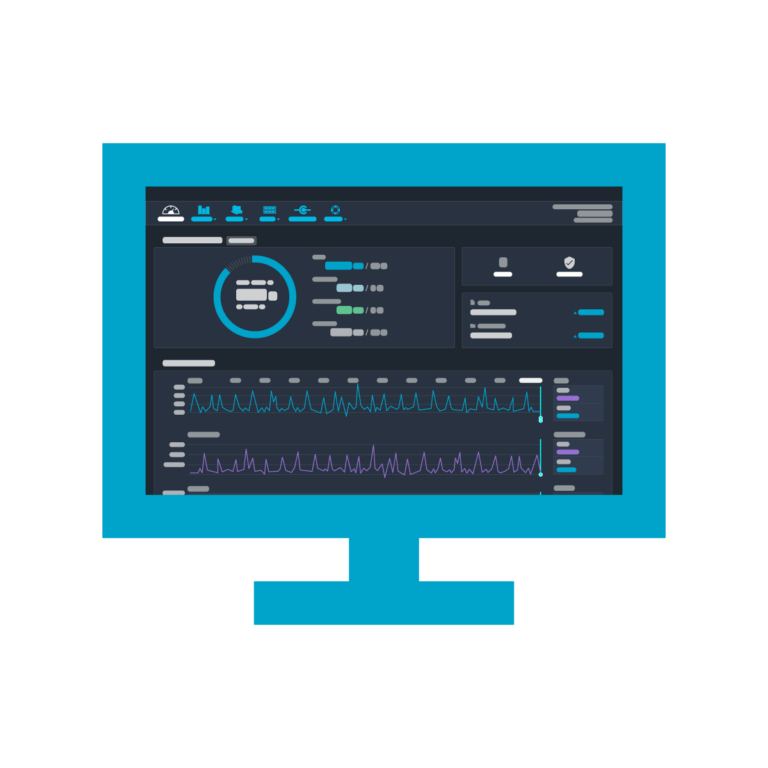
One Management Console
Qumulo Nexus provides a single, common management interface for all instances, in any location. Manage your entire data footprint through a single pane of glass.
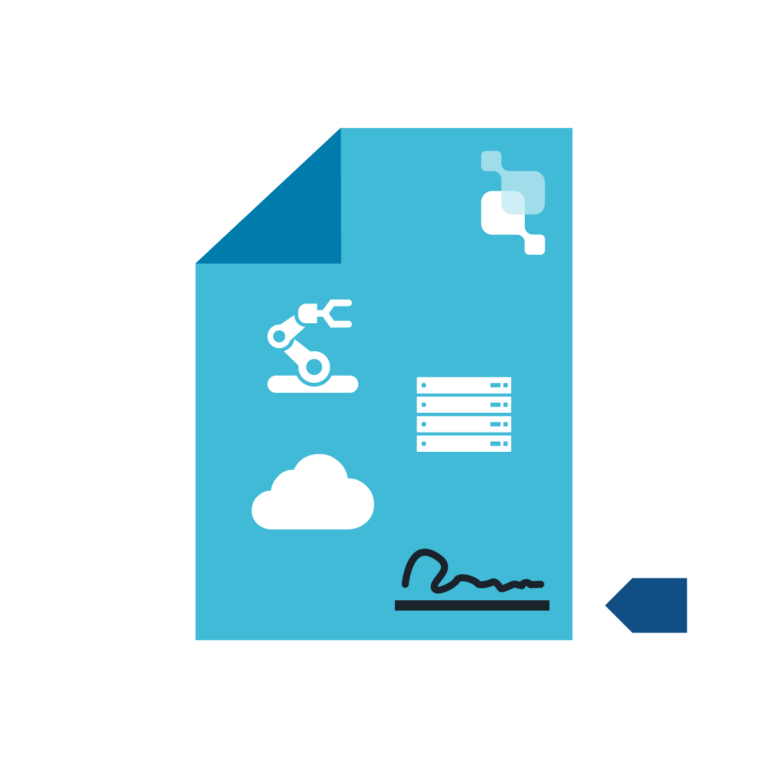
One Contract
Deploy Qumulo One simplifies data storage licensing costs for multi-site, hybrid, and multi-cloud deployments. All usage is billed on actual consumption, with no surprise fees.
What can you do with Qumulo?
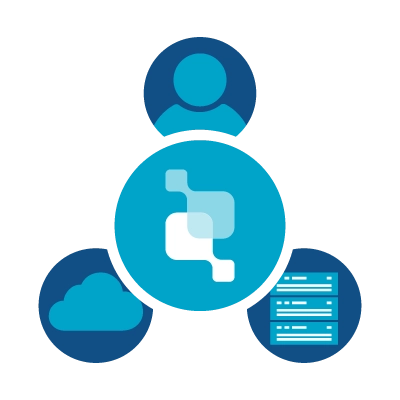
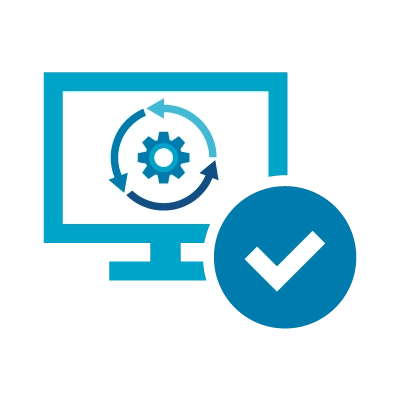
Customer Success






Where do you need to scale?
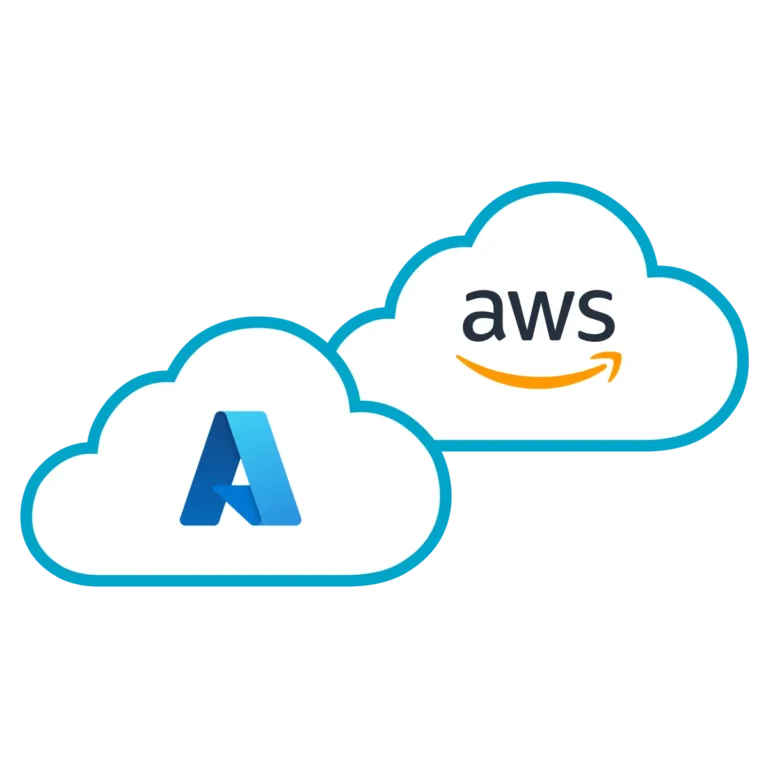
Qumulo in the Cloud
Run on your cloud of choice. Qumulo can be deployed as a managed service on Azure or self-managed on AWS.
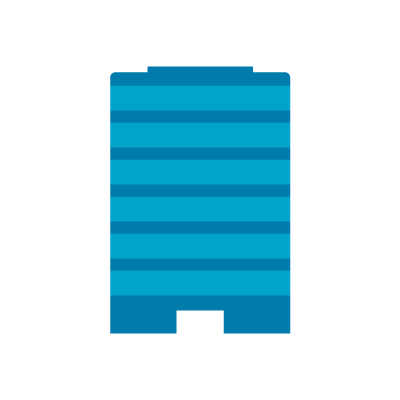
Qumulo for Data Centers
Deploy Qumulo on your preferred platform from vendors like HPE, Supermicro, and Fujitsu.
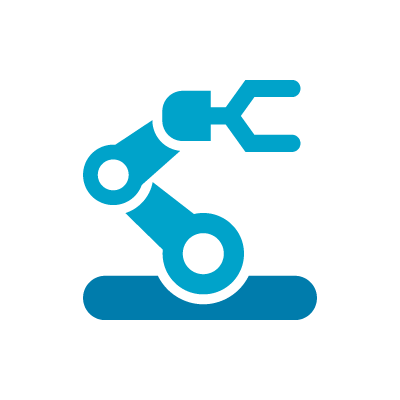
Qumulo at the Edge
Deploy at the edge to support your increasingly distributed and hybrid workloads.
Frequently Asked Questions
Imagine a single platform that can serve all your unstructured data needs, whether on-premises or in the cloud – with the same scalability and feature set on every instance. Now imagine that all those instances can be unified into a single global namespace, with all your critical data accessible to all your workloads everywhere via a universal path. Now imagine that you can manage and monitor all of them from a single pane of glass. That’s what Scale Anywhere™ means.
Besides the exabyte-plus scalability, every Qumulo instance supports multi-protocol (SMB, NFS, and object access to all shared data, snapshots, replication between Qumulo instances, replication to and from cloud object storage, quotas, and real-time system and data analytics. In other words, all the capabilities that you’d expect any on-prem NAS to have. All these features are available on all Qumulo deployments, whether on-prem or in the cloud.
You’re using your data for more workloads than ever, and you’re using it in more places, like the cloud and at the edge. You need a unified storage platform that can reach any size, run anywhere, and unify all your data across all sites and clouds in the process. Qumulo is the only unstructured data platform that can give you all of that.
The features and capabilities that Scale Anywhere delivers are included in every Qumulo deployment as part of your Qumulo license subscription.
Qumulo was founded in 2012. Since then, we’ve added over 1,000 customers in over 20 countries worldwide, who use our storage for hundreds of demanding workflows spanning healthcare, finance, energy and education. Our storage is used for the most demanding AI/ML workloads and for high-density, cost-effective long-term archives.
Yes. Whether on Azure or AWS, Qumulo’s storage architecture can scale to virtually any size, just like on-premises.
Qumulo software runs in a containerized environment in the user space of the underlying Linux appliance. Most software updates are near instantaneous and do not impact your workflows. During the software-upgrade process, the new version is deployed into a parallel container on the Linux appliance and the node is restarted using the new container. By implementing a rolling reboot of all the cluster nodes in sequence, and combining that with our support for floating IPs, your applications are minimally impacted even in the event of a full software upgrade.
We have a robust data security strategy that is multi-layered. (link to the data security section and white paper)
We offer automatic, 256-bit AES encryption of all data at rest and optional encryption in transit for SMB and NFSv4.1 clients. Our Documentation Portal provides in-depth guidance on managing encryption at rest, generating and storing ECDSA keys, and managing security keys.
Yes – Our patented snapshot technology allows you to continuously snapshot your entire dataset without incurring any treewalk penalties to determine changes. This allows us to deliver near zero RPO allowing you to continuously protect your data.
Snapshots can be scheduled at any interval, and a single Qumulo cluster can support up to 40,000 concurrent snapshots.
In our Documentation Portal, you can find deep-dive explanations about how snapshots work, how to manage snapshots by using the Web UI and qq CLI, and how to recover files by using snapshots.
Yes. We allow you to set up quotas at any level of the file system. Qumulo’s quotas take effect immediately, with no resource-intensive tree walks that can delay enforcement by hours. Nesting quotas are allowed. You can find more information on configuring and managing quotas in our Qumulo Care portal.
Yes! Qumulo supports data replication at any scale between any two Qumulo storage instances. Replication can be combined with our snapshot feature to enable rapid recovery in the event of a disaster or ransomware event, or can be leveraged to enable data pipelines that ingest data in one location, then move it to another Qumulo cluster for analysis or transformation, then to a third (and/or fourth) location for archive.
Besides large-scale replication between clusters, Qumulo also supports data replication to/from S3-compliant storage, enabling you to leverage cloud-native applications and workflows from Azure or AWS, or to archive data at scale to a cold-storage tier in the cloud. More information on replication to and from object storage is available in the Qumulo Document Portal. Additional resources on Qumulo’s replication features are available on Qumulo Care.
Qumulo’s built-in system and data analytics help organizations and administrators answer storage questions like Who? What? Where? and How much? by providing instant, real-time insight to file system capacity and activity. Capacity analytics are stored directly within the filesystem and always kept up-to-date for instant visibility without costly tree walks. Activity is displayed in real-time showing which portions of the namespace are the busiest and who is using them.
Both the filesystem and the directory structure use address spaces that can scale up to 264 entries. In theory, this supports up to 18EB of addressable storage capacity.
In practice, a single on-premises Qumulo cluster can currently scale up to 265 individual nodes.
One Qumulo cluster can support 18 quintillion files per system, 4.3 billion files per directory, and 9 exabytes max size per file. For more information, see Supported Configuration and Known Limits docs.
Qumulo includes authentication support for SAML SSO, Active Directory (including Azure Active Directory Domain Services for Azure Native Qumulo Scalable File Service deployments), and LDAP. Access to data via SMB and NFSv4.1 is managed using enterprise-standard Kerberos-based Access Control List settings.
A Qumulo cluster can be managed interactively using the intuitive Web UI, or via scripts and automated workflows built using our fully developed CLI and API-based controls. The same tools can be used on any Qumulo cluster, regardless of deployment type or location, whether in the data center or the public cloud.
The Qumulo Admin Guide covers a variety of topics, including upgrades, network management and multi tenancy, adding and replacing nodes, working with snapshots, working with file system protocols, as well as system monitoring and metrics. We are continuously adding and updating pages on the Qumulo Care portal as new features are released.
Qumulo’s Customer Success teams lead the industry in customer satisfaction by eliminating ticketing systems and escalation queues. Customers can engage with Qumulo’s support via Slack, giving them zero-latency support from our Tier-3 engineers to enable rapid diagnosis and resolution for any issue.
At Qumulo, we run hundreds of different benchmarks in our performance lab to system and performance data for all kinds of workloads. We use this data to determine the best configuration to meet each customer’s needs. Talk to your Qumulo representative or Contact Us about a solution that would work best for you.
Some legacy storage vendors protect data at the file level. If a file on the cluster is smaller than the minimum block size at the disk level (typically 4-8K per block), the system compensates by mirroring the entire file rather than using standard erasure coding protection. To ensure protection against multiple concurrent drive failures, small files may be 3x mirrored (or more). Using this approach, a 2K file on a storage system with an 8K block size may end up consuming 24K or more of cluster space.
This may not sound like much, and for a few small files, it isn’t. Even a medium-sized cluster can literally have millions of these small files, however – imagine needing to buy 1PB of capacity just to host 100TB of small-file data!
Qumulo protects data at the block layer, which means that the overhead of data protection is constant for all data regardless of file size. Qumulo eliminates the “small file tax” levied by some legacy storage vendors.
Besides our FIPS 140-2 and SOC 2 Type 2 certifications, Qumulo has also achieved HIPAA compliance for healthcare customers. More information can be found here.
Yes. A Qumulo cluster supports multi tenancy for both administration and data, with access to cluster resources (including exports shares, and cluster management protocols) being granted or denied based to specific VLANs. More information on network multi tenancy is available in both the Documentation Portal and Qumulo Care.
Among the solutions that we offer are the Qumulo OpenMetrics API (additional information in the Documentation Portal and on GitHub), the Prometheus/Grafana-based Qumulo Monitoring Dashboard (GitHub readme and repo), and the extensible Qumulo Alerts (overview, docs, repo)—we have a brand-new ‘alerts’ CLI tool coming out soon as well.
We also support management and monitoring from industry standard syslog platforms such as Splunk, ElasticSearch, and other platforms.
Qumulo Alerts provides a variety of notifications (docs) as well as default quotas and quota notifications to administrators and users (docs).
Yes! Qumulo’s support for a wide range of node hardware lets you configure the optimal balance of performance and capacity for your specific environment. Not only does this eliminate the need for tiered storage, it also eliminates the need for resource-intensive tree walks to regularly identify cold data for tiering, along with the licensing fees that some vendors charge for a tiering feature.
Software releases are issued once a month, with version updates released on a quarterly basis. Qumulo releases new features, security updates, and improvements which are included and described in the release notes. Customers can download the latest software updates from https://nexus.qumulo.com/downloads.
Yes. Qumulo integrates with the Varonis Data Security Platform, which analyzes system and security events in real time to identify potential and known threat behaviors for rapid response and containment. More information on the joint Qumulo-Varonis solution is available here.
Yes. For example, many of our customers in the commercial HPC space have teams that access data via SMB for visualization while their HPC clusters are accessing the same datasets over NFS.
Qumulo’s elegant permissions model blends POSIX, Windows/NFSv4.1 ACLs, and S3 access levels, enabling the same files or objects to be simultaneously accessed across all our supported protocols, without the risk of inadvertent access nullification from conflicting cross-protocol permissions.
Qumulo is software defined storage. We are hardware-agnostic and can run on a variety of hardware platforms. Additionally, we are continuously expanding our reach into new popular hardware vendors.
The current list of supported platforms is available in the Qumulo-Certified Hardware Guide.
Qumulo runs on a standard distribution of Linux.
As of release 6.1.1, Qumulo Core uses a hardened image of Ubuntu 20.04 Focal for the host, and Linux kernel 5.1.5 for the container image, for on-premises, cloud, and VM platforms.
We support all three of the major cloud platforms: Azure, AWS, and GCP.
Qumulo’s data-resiliency layer is built using erasure coding to ensure that data remains accessible even in the event of multiple concurrent disk failures or the loss of an entire node.
As more nodes are added, the cluster’s erasure-coding algorithm can be dynamically adjusted, lowering the overhead lost to erasure coding while still protecting against several simultaneous disk failures or node loss. While our design objective was to ensure that, at all times, MTTDL is more than 10,000 years, our MTTDL for most systems is actually closer to 100,000 years
Qumulo software runs in a containerized environment in the user space of the underlying Linux appliance. Most software updates are near instantaneous and do not impact your workflows. During the software-upgrade process, the new version is deployed into a parallel container on the Linux appliance and the node is restarted using the new container. By implementing a rolling reboot of all the cluster nodes in sequence, and combining that with our support for floating IPs, your applications are minimally impacted even in the event of a full software upgrade.
We have a robust data security strategy that is multi-layered. (link to the data security section and white paper)
We offer automatic, 256-bit AES encryption of all data at rest and optional encryption in transit for SMB and NFSv4.1 clients. Our Documentation Portal provides in-depth guidance on managing encryption at rest, generating and storing ECDSA keys, and managing security keys.
Yes – Our patented snapshot technology allows you to continuously snapshot your entire dataset without incurring any treewalk penalties to determine changes. This allows us to deliver near zero RPO allowing you to continuously protect your data.
Snapshots can be scheduled at any interval, and a single Qumulo cluster can support up to 40,000 concurrent snapshots.
In our Documentation Portal, you can find deep-dive explanations about how snapshots work, how to manage snapshots by using the Web UI and qq CLI, and how to recover files by using snapshots.
We allow you to set up quotas at any level of the file system. Qumulo’s quotas take effect immediately, with no resource-intensive tree walks that can delay enforcement by hours. Nesting quotas are allowed. You can find more information on configuring and managing quotas in our Qumulo Care portal.
Yes! Qumulo supports data replication at any scale between any two Qumulo storage instances. Replication can be combined with our snapshot feature to enable rapid recovery in the event of a disaster or ransomware event, or can be leveraged to enable data pipelines that ingest data in one location, then move it to another Qumulo cluster for analysis or transformation, then to a third (and/or fourth) location for archive.
Besides large-scale replication between clusters, Qumulo also supports data replication to/from S3-compliant storage, enabling you to leverage cloud-native applications and workflows from Azure or AWS, or to archive data at scale to a cold-storage tier in the cloud. More information on replication to and from object storage is available in the Qumulo Document Portal. Additional resources on Qumulo’s replication features are available on Qumulo Care.
Qumulo’s built-in system and data analytics help organizations and administrators answer storage questions like Who? What? Where? and How much? by providing instant, real-time insight to file system capacity and activity. Capacity analytics are stored directly within the filesystem and always kept up-to-date for instant visibility without costly tree walks. Activity is displayed in real-time showing which portions of the namespace are the busiest and who is using them.
Both the filesystem and the directory structure use address spaces that can scale up to 264 entries. In theory, this supports up to 18EB of addressable storage capacity.
In practice, a single on-premises Qumulo cluster can currently scale up to 265 individual nodes.
One Qumulo cluster can support 18 quintillion files per system, 4.3 billion files per directory, and 9 exabytes max size per file. For more information, see Supported Configuration and Known Limits docs.
Qumulo includes authentication support for SAML SSO, Active Directory (including Azure Active Directory Domain Services for Azure Native Qumulo Scalable File Service deployments), and LDAP. Access to data via SMB and NFSv4.1 is managed using enterprise-standard Kerberos-based Access Control List settings.
A Qumulo cluster can be managed interactively using the intuitive Web UI, or via scripts and automated workflows built using our fully developed CLI and API-based controls. The same tools can be used on any Qumulo cluster, regardless of deployment type or location, whether in the data center or the public cloud.
The Qumulo Admin Guide covers a variety of topics, including upgrades, network management and multi tenancy, adding and replacing nodes, working with snapshots, working with file system protocols, as well as system monitoring and metrics. We are continuously adding and updating pages on the Qumulo Care portal as new features are released.
Qumulo’s Customer Success teams lead the industry in customer satisfaction by eliminating ticketing systems and escalation queues. Customers can engage with Qumulo’s support via Slack, giving them zero-latency support from our Tier-3 engineers to enable rapid diagnosis and resolution for any issue.
At Qumulo, we run hundreds of different benchmarks in our performance lab to system and performance data for all kinds of workloads. We use this data to determine the best configuration to meet each customer’s needs. Talk to your Qumulo representative or Contact Us about a solution that would work best for you.
Some legacy storage vendors protect data at the file level. If a file on the cluster is smaller than the minimum block size at the disk level (typically 4-8K per block), the system compensates by mirroring the entire file rather than using standard erasure coding protection. To ensure protection against multiple concurrent drive failures, small files may be 3x mirrored (or more). Using this approach, a 2K file on a storage system with an 8K block size may end up consuming 24K or more of cluster space.
This may not sound like much, and for a few small files, it isn’t. Even a medium-sized cluster can literally have millions of these small files, however – imagine needing to buy 1PB of capacity just to host 100TB of small-file data!
Qumulo protects data at the block layer, which means that the overhead of data protection is constant for all data regardless of file size. Qumulo eliminates the “small file tax” levied by some legacy storage vendors.
Besides our FIPS 140-2 and Soc 2 Type 2 certifications, Qumulo has also achieved HIPAA compliance for healthcare customers. More information can be found here.
Yes. A Qumulo cluster supports multi tenancy for both administration and data, with access to cluster resources (including exports shares, and cluster management protocols) being granted or denied based to specific VLANs. More information on network multi tenancy is available in both the Documentation Portal and Qumulo Care.
Among the solutions that we offer are the Qumulo OpenMetrics API (additional information in the Documentation Portal and on GitHub), the Prometheus/Grafana-based Qumulo Monitoring Dashboard (GitHub readme and repo), and the extensible Qumulo Alerts (overview, docs, repo)—we have a brand-new ‘alerts’ CLI tool coming out soon as well.
We also support management and monitoring from industry standard syslog platforms such as Splunk, ElasticSearch, and other platforms.
Qumulo Alerts provides a variety of notifications (docs) as well as default quotas and quota notifications to administrators and users (docs).
Yes! Qumulo’s support for a wide range of node hardware lets you configure the optimal balance of performance and capacity for your specific environment. Not only does this eliminate the need for tiered storage, it also eliminates the need for resource-intensive tree walks to regularly identify cold data for tiering, along with the licensing fees that some vendors charge for a tiering feature.
Software releases are issued once a month, with version updates released on a quarterly basis. Qumulo releases new features, security updates, and improvements which are included and described in the release notes. Customers can download the latest software updates from https://nexus.qumulo.com/downloads.
Yes. Qumulo integrates with the Varonis Data Security Platform, which analyzes system and security events in real time to identify potential and known threat behaviors for rapid response and containment. More information on the joint Qumulo-Varonis solution is available here.