Technical Overview
Learn how Qumulo’s unique cloud-native architecture delivers unstructured data services for all your workloads, whether on-premises, at the edge, or in the public cloud.
Table of Contents
Table of Contents
Run Anywhere
Data services and storage management
- System management
- Web user interface
- Command Line Interface
- REST API
- Qumulo Nexus
- Access management
- Data security features
- Active Directory
- Over-the-wire encryption
- Data security features
- Authentication and access control
- Administrative security
- Domain-level admin users
- Local admin users
- Single-sign-on with multi-factor authentication
- Access tokens
- Role-based access control
- Administrative security
- Data access management
- Access Control Lists
- Kerberos enhancements
- Multi-protocol permissions support
- Object access permissions
- Management traffic restrictions
- Access Control Lists
Data Services
- Snapshots
- Snapshot locking
- Quotas
- Access logging and auditing
- Intrusion detection
- System and data analytics
- Replication
- Continuous replication
- Snapshot-based replication
The Qumulo File System
- File-system operations
- File-system scalability
- Metadata aggregation
- Qumulo Global Namespace
The Scalable Block Store
- Global transaction system
- Intelligent caching and prefetching
- Physical deployments
- Protected virtual blocks
- Software-based encryption at rest
- Cloud-based deployments
Server hardware
Download This Resource
Run Anywhere, Scale Everywhere
Qumulo’s Cloud Data Fabric lets you easily extend or integrate your applications in any environment, in any location, and on any platform. It offers the only globally-unified, platform-independent unstructured data solution that supports all your enterprise, hybrid-cloud, and multi-cloud workflows. Qumulo’s scalable file system and powerful WebUI and CLI tools make data management simple, whether you need it for your most demanding workflows or cost-effective cloud-archive storage. Our unique, cloud-native architecture frees you from the constraints of platform and place, letting you access data from anywhere, whether in your data center or in the cloud.
Our goal at Qumulo is to make unstructured data storage simple, scalable, and global for the modern enterprise. We make it simple to secure your data. We make it simple to run your most demanding workflows, whether on-premises or in the cloud. We make hybrid-cloud storage simple.
Qumulo’s Software Architecture
We’ve engineered our storage platform into a cloud-ready, scalable service that can support any file-based workflow anywhere. We’ve built a globally extensible platform. We also provide robust APIs to deliver automated management and real-time visibility into system and data usage. Our storage solutions meet the security and data protection requirements of Fortune 500 enterprises.
This page provides an overview of the architecture, components, and services of Qumulo’s unstructured data solution. It shows how our product supports a wide array of use cases, from media and entertainment to healthcare and life sciences, from cloud-based high-performance computing to cost-effective long-term cloud archive storage. We’ll also show how our unique Cloud Data Fabric can unify critical data across platforms, sites, and clouds to deliver real-time access to remote data, streamline collaboration across widely dispersed teams, and accelerate business development in virtually any industry.
Qumulo Architecture
Qumulo’s modular architecture can be abstracted into a series of layers, with specific service controls and features bundled into each layer. These layers work together to support the scalability, performance, security, and reliability of the unstructured data on a Qumulo instance, as well as the Qumulo system itself.
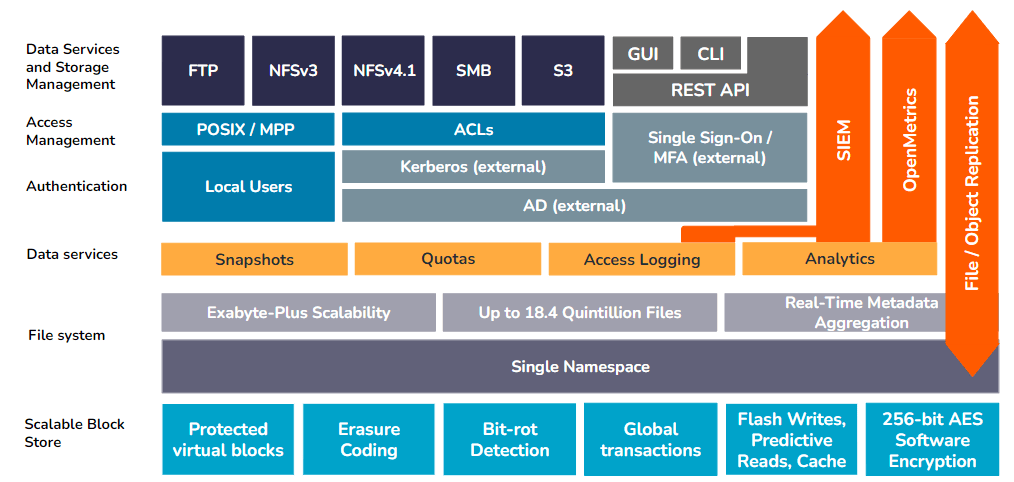
Qumulo Fundamentals
Before diving into the individual components of Qumulo’s architecture, there are several foundational principles that are important to enumerate:
- Qumulo provides a 100% software-defined distributed file system that presents a single namespace. An on-premises Qumulo cluster consists of a shared-nothing aggregation of independent nodes, each node contributing to the cluster’s overall capacity and performance. Individual nodes stay in constant coordination with each other. Any client can connect to any node and read and write across the entire namespace.
- Cloud-based Qumulo instances use object storage (either AWS S3 or Microsoft Azure Blob storage, depending on where they’re deployed) for the data layer, in which the blocks associated with any given file are abstracted and distributed across a logical collection of discrete objects.
- This cloud-native architecture eliminates the legacy relationship between compute, storage, and throughput, creating a fully elastic file storage service that can scale capacity to hundreds of petabytes, and can scale throughput beyond 100 GBps.
- With the complete disaggregation of compute and storage that Qumulo’s cloud-native architecture enables, customers have the flexibility to choose the specific levels of throughput and capacity they need, independently of one another. A customer can even deploy a Qumulo instance with an initially-low compute footprint, then temporarily scale the service’s compute allocation to dramatically increase throughput for a brief period of time, then scale it back down again afterward without at any time needing to deploy additional capacity.
- Qumulo is engineered for scale. We ensure all aspects of our product can comfortably support petabytes to exabytes of data, trillions of files, millions of operations, and thousands of users across dozens of sites, regions, and deployments.
- Qumulo is engineered for geographic distribution, enabling widely dispersed teams to collaborate on shared datasets without risk of data corruption or loss.
- Qumulo is self-optimizing for maximum performance. Every Qumulo instance tracks data access using a heat map to identify frequently-accessed data blocks. These blocks are proactively moved by an internal prefetch algorithm: data blocks on long-term storage media are moved to flash storage as their heat score increases. If the heat score continues to rise, data that is already on flash storage is proactively moved to system memory for even faster access. At a global level, across all Qumulo instances for all Qumulo customers, the cache hit rate is ~95% of all read requests.
- Qumulo is highly available and strictly consistent, built to withstand component failures in the infrastructure while still providing reliable service to clients. We do this through the use of software abstraction, erasure coding, advanced networking technologies, and rigorous testing. When data is written to Qumulo’s file system, the write operation is not confirmed to the service, user, or client until the data has been written to persistent storage. Thus any subsequent read request will result in a coherent view of the data (as opposed to eventually consistent models).
- Qumulo delivers platform-agnostic file services for the public, private, and hybrid cloud. Qumulo’s software makes few assumptions about the platform on which it runs. It abstracts the underlying physical or virtual hardware resources in order to take advantage of the best public and private cloud infrastructure. This enables us to leverage the rapid innovation in compute, networking, and storage technologies driven by the cloud providers and the ecosystem of component manufacturers.
- The Qumulo management model is API-first. Every capability built by Qumulo is first developed as an API endpoint. We then present a curated set of those endpoints in our command line interface (CLI) and the WebUI, our visual interface. This includes system creation, data management, performance and capacity analytics, authentication, and data accessibility.
- Qumulo ships new software rapidly and regularly. We release new versions of our software every few weeks. This enables us to rapidly respond to customer feedback, drive constant improvement in our product, and insist on production-quality code from our teams.
- Qumulo’s container-based architecture enables a unique upgrade process that minimizes disruption to users and workflows. On a rolling, node-by-node basis, the new operating software is deployed in a parallel container to the old version. Once the new instance has initialized, the old environment is gracefully shut down, and the upgrade proceeds to the next node until the entire cluster has been upgraded.
- Qumulo’s Customer Success team is highly responsive, connected, and agile. Qumulo has the ability to monitor every Qumulo deployment remotely via our cloud-based Mission Qontrol service, which lets us passively track storage and service telemetry (We do not have the ability to view or access file-system data on any Qumulo customer deployments). Our customer success team uses that data to help customers through incidents, to provide insight into product usage, and to alert customers when their systems are experiencing component failures. This combination of intelligent support and rapid product innovation powers an industry-leading NPS score of 80+.
The Qumulo File System
All unstructured data stored on a Qumulo file system is organized into a single namespace. This namespace is POSIX-compliant to support NFS3 clients, while also supporting the Access Control List standard used by the NFSv4.1 and SMB protocols.
Qumulo stands out due to several key features: its ability to scale a single namespace to virtually any size, the seamless integration of system and data analytics into file system operations, support for S3 and traditional protocols like NFS and SMB, and its innovative approach to managing multi-protocol permissions.
File-system operations
Qumulo’s file system was engineered from the beginning to seamlessly scale to exabyte-plus capacity in a single namespace that can host trillions of files that can be shared via standard NFS and SMB protocols. Additionally, the file system was architected with the ability to efficiently monitor file-system updates and actions, and to aggregate metadata-based statistics and operations, enabling real-time system and data analytics without resorting to resource-intensive, time-consuming tree walks.
File-system scalability
A single Qumulo instance can scale to exabytes of capacity and 264 nodes (~18.4 quintillion files) without any of the problems common to other platforms, such as inode depletion, performance slowdowns, and long recovery times after component failures.
Metadata aggregation
Qumulo’s unique architecture tracks metadata in real time as files and directories are created or modified. Different metadata fields are summarized to create a virtual index. As changes occur, new aggregated metadata is gathered and propagated from the individual files to the root of the file system. Every file and directory operation is accounted for, and the resulting changes are immediately merged into the system’s analytics. The results, aggregated across the entire file system, are made visible through Qumulo’s built-in analytics engine, delivering actionable data visibility without requiring expensive file data platform tree walks.
The Scalable Block Store
Beneath the Qumulo file system is a protected, modular layer that serves as the interface between potentially billions (or more) of files and directories and the physical data medium on which they’re stored. In the Qumulo modular architecture, the Scalable Block Store layer fills this role.
Global transaction system
Since Qumulo uses a distributed, shared-nothing architecture that makes immediate consistency guarantees, every node in the service needs to have a globally consistent view of all data at all times. The Scalable Block Store leverages a global transactional approach to ensure that when a write operation involves more than one block, the operation will either write all the relevant blocks or none of them. For optimum performance, the system maximizes parallelism and distributed computing while also maintaining the transactional consistency of I/O operations.
This approach minimizes the amount of locking required for transactional I/O operations and enables any Qumulo deployment to scale to many hundreds of nodes.
NeuralCache and intelligent prefetching
Qumulo’s software incorporates a number of inherent features and configurable controls, all designed to protect the data on the cluster.
- All metadata, which is the most often read in any data set, resides permanently on the storage instance’s flash tier.
- Frequently-read (as measured by a proprietary “heat index”) virtual blocks are stored on flash, while virtual blocks that are read infrequently are moved to colder media, i.e the system’s HDD tier (if available).
- As data is read, the Qumulo instance monitors client behavior and intelligently prefetches new data into system memory on the node closest to the client in order to speed up access times.
Physical Qumulo deployments (clusters)
On a physical Qumulo cluster, the Scalable Block Store serves as the interface between the file system and the underlying storage media, which can be either solid-state flash devices (SSDs) or hard disk drives (HDDs). This layer is primarily responsible for guaranteeing data consistency across all nodes in a physical cluster, ensuring optimal performance for both read and write requests, and for providing data security, integrity, and resiliency against component failure.
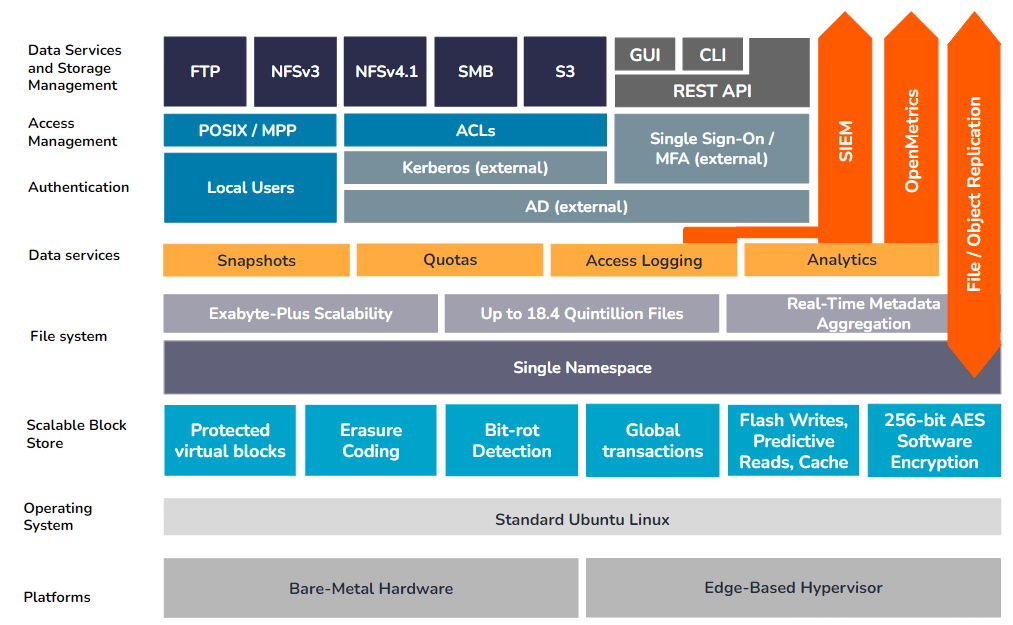
Protected virtual blocks
The storage capacity of a physical Qumulo cluster is conceptually organized into a protected virtual address space. Each address within that space stores either a 4K block of data or a 4K erasure-coding hash that can be used to rebuild any data blocks lost to hardware failure. The ratio of data blocks to erasure-coding blocks is determined by the size of the physical cluster – as more nodes are added, the ratio adjusts to provide greater overall efficiency while still protecting against both disk and node failure.
In addition to the protection offered by erasure coding, the virtual block system also includes a bit-rot detection algorithm to protect against on-disk data corruption.
Cloud-based Qumulo instances
For Qumulo instances deployed on Azure, many of the functions provided on-premises by the Scalable Block Storage layer, such as on-disk encryption, erasure coding, bit-rot detection, and block management, are provided as core features of the underlying Azure Blob Storage service.

Encryption at rest
On physical Qumulo clusters, the Scalable Block Store includes an AES 256-bit software-based algorithm that encrypts all file system data before writing it to the data layer. This algorithm initializes as part of the initial cluster build process, and compasses all file system data and metadata at the block level for the entire lifespan of the cluster.
Qumulo clusters in the cloud rely on block-level encryption on the underlying object-storage layer, implemented and maintained by the cloud service provider. This ensures that all at-rest data on any Qumulo cloud-based instance is fully encrypted.
For enterprises that require it, the built-in Qumulo encryption algorithm used in every physical cluster is compliant with FIPS 140-2 standards, as are the encryption services provided by both Azure and AWS object storage.
Data Services
The Data Services layer includes five management features: snapshots, replication, quotas, access logging and auditing, as well as system and data analytics.
Snapshots
Snapshots on a Qumulo cluster can be used in several ways to protect the cluster’s data:
- They can be used locally for quick and efficient data protection and recovery.
- A snapshot of the live data on one Qumulo cluster can be replicated to a secondary Qumulo instance, such as an Azure Native Qumulo Cold service instance, which could support an immediate failover of file data services in the event of a systemic outage in the primary location.
- Qumulo snapshots can also be paired with third-party backup software to provide effective long-term protection (with more robust version control for changed files) against data loss.
Snapshot Locking
To provide added protection against ransomware attacks or premature deletion of critical snapshots via a compromised administrator account, snapshots can be cryptographically “locked”, preventing the alteration or premature deletion of a snapshot even by an administrative user.
The use of locked snapshots requires an asymmetric cryptographic key pair, with the public key installed directly on the Qumulo instance and the private key stored externally according to the organization’s own established key-management practices.
Quotas
Quotas enable users to control the growth of any subset of a Qumulo namespace. Quotas act as independent limits on the size of any directory, preventing data growth when the capacity limit is reached. Unlike with other platforms and services, Qumulo quotas take effect instantaneously, enabling administrators to identify rogue workloads via our real-time capacity analytics and instantly stop runaway capacity usage. Quotas even follow the portion of the namespace they cover when directories are moved or renamed.
Access logging and auditing
Audit logging provides a mechanism for tracking Qumulo file-system events and management operations. As connected clients issue requests to the cluster, event-log messages describe each attempted operation. These log messages are then sent over the network to a designated remote syslog instance, e.g., an industry-standard Security Information and Event Management (SIEM) platform such as Splunk.
Real-time intrusion and ransomware detection
Qumulo has partnered with third-party providers Superna and Varonis to enable real-time monitoring of event and access logs to identify and respond to cyberattacks. To learn more about Varonis with our Azure Native Qumulo solution, visit our Varonis Integration with ANQ page. Information on Superna Ransomware Defender is available here.
Replication
Qumulo’s built-in replication service can copy data at scale between any two Qumulo storage instances. Besides protecting data against cyberattacks, a secondary location with another Qumulo cluster can also serve as failover storage in the event of a site-level outage.
Since all Qumulo instances support the same replication features and deliver the same services regardless of location, replication can be configured to run in any direction between any two Qumulo endpoints, whether on-premises, in AWS, or on Azure.
Continuous replication
This form of replication simply takes a snapshot of the data on the source Qumulo cluster and copies it to a directory on a target cluster. As long as the replication relationship is active, the system scans any modified files to identify and copy only the specific changes to the target, overwriting any previous versions of the data.
Snapshot-based replication
With snapshot-based replication, snapshots are also taken of the target directory on the secondary cluster. Once a replication job has been completed, a new snapshot of the target directory is created, ensuring data consistency across both clusters, while also maintaining a change log and version history for each file on the target.
System and data analytics
Qumulo’s software stack is engineered to offer real-time insight into system and service metrics, including capacity and performance, in every Qumulo instance. This enables customers to troubleshoot applications, manage capacity consumption, and plan expansion (or archive) strategies. Qumulo’s analytics are powered by aggregating metadata changes across the file system as they happen.
The web interface includes real-time monitoring tools for tracking system performance, capacity usage, and current activity on the local Qumulo instance. For enterprises who wish to export this information to an external monitoring solution, Qumulo supports the OpenMetrics API standard for exporting and compiling syslog data.
Instance and data service management
As an industry-standard file-data storage service, Qumulo supports all unstructured data-access protocols: SMB, NFS, and NFSv4.1. It also supports object access using the S3 protocol standard. Qumulo also supports FTP and REST API access to select data types.
System management
Any Qumulo instance, whether on-premises or in the cloud, can be managed using the same standard tools: a built-in web user interface for interactive storage and data management, a CLI-based command library, or an API-based set of management tools.
Web user interface
Qumulo offers a browser-based management interface, accessed from the storage instance itself with no separate VM or service needed. The intuitive Web UI is organized around six top-level navigation sections: Dashboard, Analytics, Sharing, Cluster, API & Tools, and Support.
Command Line Interface (CLI)
The Qumulo CLI supports most (but not all) of the API library and is focused on system administration. The CLI offers a scriptable interaction method for working with a Qumulo instance. A full list of commands can be found in our Knowledge Base (care.qumulo.com).
REST API
The REST API is a superset of all capabilities in the Qumulo data platform. From the API, administrators can:
- Create a namespace
- Configure all aspects of a system (from security, such as identity services and system management roles, to data management and protection, including quotas, snapshot policies, and / or data replication)
- Gather information about the target Qumulo instance (including capacity utilization and performance hotspots)
- Access data (including read and write operations)
- The API is “self-documenting,” making it easy for developers and administrators to explore each endpoint (and see example outputs). Qumulo maintains a collection of sample uses of our API on GitHub (https://qumulo.github.io/).
Qumulo Nexus
As Qumulo customers increasingly move to multi-cloud, multi-site enterprise operations, they need to reduce the complexity of monitoring each Qumulo instance’s availability and service metrics through separate management interfaces. With Qumulo Nexus, customers can consolidate monitoring operations for all their Qumulo instances – whether on-prem, at the edge, or in the cloud – under a single management portal that delivers the same real-time analytics and data visibility as the local web interface.
Security and access management
Qumulo’s software incorporates several inherent features and configurable controls, all designed to protect access to the data on the cluster.
Qumulo data security features
Every Qumulo instance, whether on-prem or in the cloud, leverages a pair of controls that ensure that all data within the file system is secured against corruption, loss, or intrusion at the data storage level.
Active Directory integration
Qumulo’s security access model was engineered to leverage Microsoft Active Directory (AD) for both administrative and user rights and permissions. Besides the obvious benefits of having a single source of record for all user accounts, the use of AD for both privilege and permissions management supports industry best practices for the following:
- Seamless integration with Kerberos-based authentication and identity management protocols
- Integration with SSO and MFA access providers
- The use of Access-Control List-based permissions for SMB and NFSv4.1 clients to file system data
Over-the-wire-data encryption
Even with the appropriate share and data-level security settings in place, some enterprises need an additional layer of data security to protect data from unauthorized access. For those environments, Qumulo also supports over-the-wire data encryption to and from supported clients.
For SMB3 shares, Qumulo supports both cluster-wide and per-share encryption when needed. NFSv4.1 exports that require enhanced security can be configured to use either krb5i packet signatures that ensure data integrity or krb5p-based packet encryption to prevent interception during transit.
All object-based traffic is automatically encrypted using standard TLS / HTTPS encryption standards.
Authentication and Access Control
Access to data in the Qumulo file system, as well as access to the Qumulo storage system, use industry-standard authentication and access protocols, ensuring enterprise-grade access management, identity control, and auditability.
Administrative Security
System-level rights and privileges are granted based on membership in one or more local groups on the individual Qumulo instance. Administrative rights are granted to all local and domain accounts that are members of the cluster’s built-in Administrators group.
Domain-level administrative users
Most enterprise security policies require that the administration and management of critical enterprise systems follow a one-user, one-account policy to ensure accurate records of system access and privilege use. The simplest method for complying with this policy is by adding the relevant Active Directory user accounts to the cluster’s local Administrators group.
Local administrative users
Every Qumulo instance comes with a default account, called admin, which is automatically assigned membership in the local Administrators group and has full administrative rights and privileges to the cluster.
Single sign-on with multi-factor authentication
Single sign-on (SSO) eliminates the need for an administrator to re-enter their login credentials to gain access to the system. Enterprises want SSO not just because it streamlines the login process, making it more convenient for admins to authenticate, but also because it reduces the risk of account theft via keystroke loggers or interception as the login attempt traverses the network.
Multiple-factor authentication (MFA) adds another layer of security to the login process. It requires that admin users retrieve a one-time code from either a key token or a challenge request on a separate device, neither of which would be in the possession of an intruder.
Qumulo’s SSO solution integrates with Active Directory via Security Assertion Markup Language (SAML) 2.0. For MFA, customers can leverage any Identity Provider (IdP) that integrates with the AD domain registered on the cluster, including but not limited to OneLogin, Okta, Duo, and Azure AD.
Access tokens
To simplify the process of automated storage and data management via Qumulo’s API functionality, Qumulo offers administrators the option of generating a long-lived API token that can be used indefinitely by automated workflows until the key is either revoked or deleted. The token is generated by an administrator via CLI, and can be attached to each API-based workflow, which can now make authenticated API calls without having to log in.
For auditing purposes, each token maps to a specific AD or cluster account. If the associated user account is deleted or deactivated, the access token will stop functioning.
Role-based access control
Role-based access control (RBAC) allows administrators to assign fine-grained privileges to non-administrative users or groups who require elevated rights to the cluster for specific management tasks. The use of the RBAC model allows the secure delegation of privileges on an as-needed basis without needing to confer full administrative rights. It also enables enterprises to grant necessary system privileges while ensuring a verifiable audit trail of access and privilege use.
Data access management
Qumulo uses the same security model for managing access to file system data, using enterprise standard practices, protocols, and tools to manage and track access to all files and directories on the system.
Access Control Lists
For workloads shared via SMB and NFSv4, Qumulo supports authentication via Active Directory and Windows-style Access Control Lists (ACLs) that can be shared across both protocols.
Kerberos enhancements
All SMB and NFSv4.1 data requests, if originating from a Windows or Linux client that is joined to the same domain as the Qumulo cluster (or joined to a trusted domain), are authenticated using Kerberos-based user identity management.
Multi-protocol permissions support
Qumulo supports making the same data on the file system available over multiple protocols simultaneously. In many cases, an SMB share on the cluster may also be configured as an NFSv3 export, an NFSv4.1 export, and an object storage container. While this maximizes the cluster’s flexibility, there are some considerations that need to be taken into account when it comes to managing permissions.
SMB and NFSv4.1 both use the same ACL-based permissions model, in which access is granted or denied to the user by virtue of the user’s Active Directory account’s membership in one or more groups whose access has been configured at the data level.
For mixed SMB/NFSv3 workloads, however, there can be a mismatch between a given file or directory’s ACL permissions and its POSIX settings. A Qumulo instance can be configured for mixed-mode operations, in which SMB and POSIX permissions are maintained separately for files and directories that are shared across both protocols.
For mixed-protocol workloads, Qumulo’s proprietary multi-protocol permissions (MPP) model preserves SMB ACLs and inheritance even if the NFS permissions are modified.
Object access permissions
If a directory on the cluster is shared via the S3 protocol, it is treated as an S3 bucket, and all subdirectories and files within that directory are treated as objects within the bucket.
When a user or workflow attempts to access an object, the system uses the access key provided by the client to identify the key’s mapped Active Directory or local user ID, and then checks that ID against the object’s SMB / NFSv4.1 access control list.
Management traffic restrictions
In addition to using SSO and MFA-based authentication of designated administrative accounts, Qumulo also supports security policies that require the restriction of admin-level access to specifically designated networks or VLANs by offering the ability to block specific TCP ports at an individual VLAN level.
In this manner, a Qumulo instance can be configured to segment management traffic – e.g., API, SSH, web UI, and replication traffic – from client traffic, e.g., SMB, NFS, and object access.
Cloud Data Fabric
In an interconnected, multi-cloud world, geographically dispersed teams applications and teams need the ability to collaborate on shared datasets without the latency of a WAN connection or the long wait to replicate data at scale between sites.
Qumulo’s Cloud Data Fabric (CDF) extends Qumulo’s scalable file system across two or more Qumulo instances, enabling geographically-dispersed teams and workflows to share a single dataset in real time. CDF not only delivers low-latency access to critical data between on-premises, edge, and cloud Qumulo instances; in many cases, it entirely eliminates the need for large-scale replication in shared workflows.
CDF unifies one or more shared datasets across sites, clouds, platforms, and geographies, making data available to teams, applications, and organizations that need to collaborate in real time. The use of CDF lets enterprises minimize WAN traffic while simplifying cross-site data and security management.
Global Namespace
Qumulo’s Cloud Data Fabric enables a truly global namespace in which critical datasets are shared between Qumulo instances everywhere: in the data center, in the cloud, and at the edge. Qumulo’s Global Namespace uses uniquely innovative features that combine to enable real-time data visibility and worldwide collaboration on shared data.
Metadata synchronization
Instead of synchronizing file and folder data immediately across all instances that share a given dataset, Qumulo’s Global Namespace implementation initially replicates only the metadata between Qumulo endpoints, allowing remote clients to see the entire file and folder structure of remote datasets with only a few milliseconds’ delay.
Distributed locking
To ensure data integrity in a distributed environment where any file can be modified by any user or application across multiple locations, CDF uses a locking algorithm that coordinates file access across all endpoints. This ensures that every data transaction is authorized, logged, and strictly consistent with all prior actions.
Data security
Replicating file-system metadata between endpoints also replicates the ACLs (SMB and NFSv4.1) and POSIX permissions. If all users across the portal share the same authentication source, data access is automatically carried over between endpoints.
If a portal is deleted, the links to all spoke endpoints are terminated, and all locally cached data is deleted from every spoke. Unless the underlying share or export is also deleted, the data remains on the Qumulo instance that served as the hub endpoint.
Data Portals
Cloud Data Fabric’s unique approach to unified data access is based on data portals. Portals are published at the level of the individual SMB share or NFS export (or both, since Qumulo also offers multi-protocol data sharing). The creation of a CDF portal makes the data within that share or export available to one or more additional Qumulo instances.
CDF portals can support both read-only and bidirectional use cases.
Portal endpoints
Cloud Data Fabric portals are built using a hub-and-spoke topology, in which one Qumulo instance (the “hub”) serves as the authoritative owner of the portal data and coordinates all data-access operations across all portal endpoints. Each portal has a single hub, with one or more “spoke” endpoints acting as extensions of the portal’s namespace.
To create a new portal, an administrator identifies the specific share or export on the hub to be published, assigns at least one additional Qumulo endpoint as a spoke, and designates the new portal as either read-only or bidirectional. Additional Qumulo instances can be added to the portal as spokes as appropriate.
Each endpoint in a CDF portal may also function as an independent Qumulo storage instance, hosting additional shares and / or exports restricted to use by local clients only.
Journaled logging
All file-system operations within a given portal are tracked via a distributed logging mechanism. Each data access event (create, read, modify, delete, etc.) is recorded in the shared log and replicated to all the other endpoints across the portal.
Logical time tracking
The use of a shared logical time offset to coordinate events across endpoints, rather than an absolute physical timestamp, ensures that data transactions are applied in the correct order across all nodes in all endpoints without having to reconcile time zone differences or time offsets between the different Qumulo instances.
Logical logging and recovery
If a portal spoke loses connectivity to the hub, portal data is unavailable to any of that spoke’s clients. Across the rest of the portal’s endpoints, the distributed log file continues to track changes to data within the portal.
Upon reconnection, the affected spoke will replay events in the log in their proper sequence to reconcile its locally cached data with the rest of the endpoints in the portal.
Transfer optimization
Cloud Data Fabric has been engineered to make the most of limited bandwidth, delivering high-performance, low-latency access to remote data at scale, even in widely distributed enterprises. It does this by waiting for clients to request the specific portal data they need, caching only relevant data locally on each endpoint, and replicating only changed data blocks between endpoints rather than entire files or whole datasets.
Local caching
The first access of data from a remote endpoint causes the requested file or folder to be “pulled” across the network and cached on the local Qumulo endpoint. Assuming no changes to the data after the first access, all subsequent access requests for the same data will be served from the local cache.
Sparse file systems
Each spoke builds a shadow file system, independent of any other file data that it hosts locally, containing the portal’s metadata as well as any locally cached portal data. The local instance uses a heat map, similar to but independent of the intelligent caching system that optimizes read performance for the local file system, to monitor the frequency with which cached data is accessed.
The size of this sparse file system varies with the specific Qumulo instance’s available storage capacity. As new portal data is requested and the sparse file system fills up, older data is flushed from the cache to ensure optimal performance for new incoming data.
Predictive Caching
As files and folders are accessed by local clients and applications, each endpoint monitors what portal data is passively added to the sparse file system cache over time and begins to identify patterns in client and application behavior. CDF uses these access patterns to anticipate which data blocks are likely to be requested next and pre-emptively prefetches those blocks into the sparse file-system cache before they are requested.
Server hardware
Qumulo’s software runs on virtually any standard, enterprise-grade x86-64-based hardware, although customers looking for optimal availability and performance should consult with Qumulo directly before choosing the appropriate hardware configuration.
The underlying Linux operating system is locked down, allowing only the operations needed to perform the required supporting tasks of the Qumulo software environment. Other standard Linux services have been disabled in order to further reduce the risk surface for an attack.
Fully native software stack
Although Linux includes open-source components to provide both NFS and SMB client and server services (e.g. Samba, Ganesha, etc.), these services are not included in the hardened Ubuntu image that supports the Qumulo software environment. Qumulo develops and controls all code used for data-access protocols NFS, SMB, FTP, and S3 – in the Qumulo operating environment.
Instant upgrades
Qumulo’s iterative development process is simple and streamlined, with new software updates released regularly. This enables rapid innovation to develop and roll out new features and fosters a more secure storage platform.
Qumulo engineered the upgrade process to be quick and easy. The Qumulo Core software stack is fully containerized, enabling the upgrade of an entire cluster in 20 seconds, regardless of size. The need for rollbacks is eliminated since the functionality and stability of the updated version can be fully validated before the older version is shut down.